We collectively produce massive amounts of data each day, whether we are aware of it or not. Social interactions on the internet, bank transactions, videos watched, online searches or purchases, IoT devices, all create data points.
In 2020, humans and computers generated more than 64 zettabytes of data (a size unit hardly anybody knows). That’s equivalent to 64 trillion gigabytes (or in layman’s terms, 4 billion of today’s largest capacity disk drives). With this largely disorganised treasure trove of information, big data analytics can drive important decisions. You can use the output of these decisions to improve processes and policies, and create customer-centric products, services, and experiences.
Throughout the course of this article, we’ll explore big data in closer detail. We will focus most of our attention on learning technology big data, as that is where our expertise lies. However, much of what we mention here still holds true across other verticals.
Are you ready to set sail on a seemingly endless sea of data?
What is Big Data
As the name would suggest, big data designates a very large amount of data. However, this appellation does not limit itself to volume. To qualify as being big data, the data must possess at least the five following characteristics:
1. Volume
While it is true that volume is not the only characteristic that makes big data “big”, it is certainly one of its primary features. Data is generated from countless sources and in different formats. As a result, you end up with a far-reaching goldmine of information begging to be extracted and analysed.
2. Velocity
Velocity refers to the high speed generation of data. On average, every person creates 1.7 megabytes of data every second. High velocity generation of data is so fast that it requires specific (distributed) processing to be effective and in real time.
Storing data into a traditional structured database system before analysis is not required any more. Additionally, the analysis process sources the data wherever it is located. An example of data generated with high velocity would be email or social media posts. This is not because you’re a super speedy social poster. It’s because so many of us are cooking up new social media posts at the same time!
3. Variety
Big data is sourced from a great variety of origins which include structured, unstructured, and semi-structured data. This requires different processing capabilities and specific algorithms for each set of data and structure type. For example, variety is present when simultaneously analysing data from emails, social interactions, LMS history records, and customer feedback.
3.1 Structured Data
Structured data includes quantitative values or defined values (such as single, married, separated, etc.) data stored in rows and columns. Furthermore, you can map its elements into fixed predefined fields. This is how you would typically structure an Excel spreadsheet as a table of data. Structured data follows a model a database designer has created. For example a list of sales by region, product, or customer.
Using this structure makes it easier to link data tables to form relations and cross-reference data. For instance, you can view sales history by region and link it to when your salespeople completed eLearning content to prove the value of your training initiatives.
Ultimately, it is easy to store, analyse, and search structured data using Structured Query Language (SQL). It is estimated that around 20% of all data that exists today is structured. Common examples of structured data include financial data, login counts, and demographic details.
3.2 Unstructured Data
Unstructured data includes qualitative data that lacks any predefined structure. As such, it cannot be easily captured in a standard row-column relational database. Fortunately, with the use of text analysis software, you can scan through and extract valuable information from unstructured data.
Examples of unstructured data consist of social media posts, images, and open-ended customer feedback. This type of data is very difficult to analyse directly using standard SQL.
3.3 Semi-Structured Data
As you have correctly guessed, semi-structured data is a hybrid of structured and unstructured data. Emails are a good example of semi-structured data. They include organised data like the sender, recipient, subject and date, as well as unstructured data in the body of the message.
4. Veracity
Veracity is the quality and credibility of the data. Big data is only valuable if it is accurate. Is the data point coming from a trustworthy source which generated the data in good faith? Is the dataset representative of the whole picture of what is being analysed or is it skewed?
Both quality and accuracy are sometimes difficult to control when it comes to collecting big data. After all, big data contains a multitude of data variables from different sources.
Gathered data always comes with inconsistencies, uncertainties and outliers. Within structured data, typos and convention errors are the usual culprits. For instance, when filling in a form, one person may type ‘Growth Engineering’ in the company name field, and another ‘GROWTH ENGINEERING LTD’. Both instances will be seen as different names, even though they both refer to the same entity.
At the same time, unstructured data comes with a whole new set of veracity issues. Social noise, human bias, and data source issues can impact the quality of the data.
Good practices to improve data processing begin with establishing the validity of your data.
5. Value
Value refers to the usefulness of the data collected for your business. Data by itself, regardless of how much of it you have, usually isn’t very useful. To be valuable, you need to convert it into insights or information.
At this stage data processing plays an important role. By using adequate processing logic, you can derive useful insights from an endless sea of data. By doing so, you add value to your decision making process. A study by BARC reported that organisations are now reaping the benefits of big data. For instance, organisations reported:
- 69% better strategic decisions
- 54% enhanced operational processes
- 52% better understanding of customers
- And a 47% effective cost reduction
Even though companies have been collecting huge amounts of data for decades, the concept of big data only gained popularity in the mid-2000s. Enterprises finally realised that this data had value and that using it effectively had huge potential for their business.
The Role of Big Data in eLearning
In the context of learning technology, big data is the data generated when a user or admin interacts with a Learning Management System (LMS), LXP, or mobile app solution. For instance, when employees complete learning objects, their progress, results and any additional data created and collected during the module is considered to be part of “big data”.
Collecting big data from your learning technology solution is similar to sensitive intelligence gathering in a successful military operation. As mentioned previously, all actions performed in an LMS generate data.
Successful military operations result from gathering, analysing and decoding data, and effectively turning it into intelligence. Likewise, successful eLearning interventions require admins and L&D professionals to derive insights from the big data they have.
To be more specific, big data can help learning professionals to:
- Gain actionable insight on learning and knowledge gaps across the organisation
- Identify preferred methods of conducting learning events for individuals or for groups
- Highlight the strengths and weaknesses in an organisation’s learning strategy
- And understand how to personalise a learning journey to improve the learner experience and results
The Benefits of Big Data Analytics for L&D Teams
Now that we have discussed the role of big data in eLearning, let’s take a closer look at how L&D teams can benefit from analysing big data.
Personalised Learner Experiences
Analysing your data helps you improve the training you provide. It gives L&D teams the information needed to identify what is working and what is not. For instance, you are able to see:
- Where learners have difficulties and spend more time
- Where learners get stuck
- The time of the day they learn best
- The type of devices they use to access the content
- As well as which modules or links get shared the most
With all of this data, you can revise learning initiatives to match your learners’ needs and preferred styles. As a result, you can deliver more personalised learner experiences, and increase the effectiveness and speed of your training.
Return On Investment
Big data analytics first increases return on training investment by increasing training efficiency. With it you can pinpoint which learning objects are not producing the desired change in behaviour and learning outcomes. This then allows you to implement fast improvements and measure their effectiveness ongoing.
Furthermore, L&D teams can revise or remove content which learners are struggling to complete. This helps make their learning easier and eases their progress through the training material.
Secondly, big data analytics points out which training initiatives are not producing the expected business results. Such ineffective initiatives adversely impact your business ROI. With big data, however, you can link specific training interventions to increased revenue, productivity, reduced risk, etc., and make high-impact recommendations to users.
Shortened Refresh Cycle
Big data enables you to make better decisions faster than ever before. In fact, big data is created in real time. You receive it immediately upon each interaction. As such, L&D teams can start analysing employee performance after each group of learners have finished a course. They can then implement any necessary changes right away.
Prediction Capabilities
Based on the patterns you extract from big data, you have the power to predict where learners might struggle or perform. With this knowledge, you can develop courses that give your learners the best chance of achieving good results. Alternatively, you can provide additional levels of support throughout sections of your training that you know will be “tougher”, to help your learners across the finishing line.
Furthermore, big data can give you an idea of the skills present within your organisation. This allows you to anticipate and address skill gaps.
Target Effective Learning Strategies
Big data also gives a complete overview of which learning strategies are most effective. It helps identify strategies that work and those that don’t. For instance, the language-learning app Duolingo used big data from its learners to determine which learning sequences led to the best outcomes.
In turn this helps you better allocate your budget. And if you’re spending your money in a wiser fashion you’re more likely to achieve your organisational goals.
Improves Competitiveness
The insights gained from big data analytics helps you save money, improve competency and performance, build marketing and production capabilities, capture more customers and close more sales. Ultimately, this helps you better meet your customer’s needs and gain more bargaining power.
Without the right data, making the right decisions is a shot in the dark. Getting it right through trial and error can be too time-consuming and too costly to pull off. But not making data-informed decisions is a costly business in itself.
Best Practices to Effectively Manage and Analyse Big Data
Implementing big data analytics within your learning technology allows you to improve your learning strategy and user experience. But what’s the best way to collect, manage and analyse your learning data?
There are seven indispensable processes that will help make the most out of big data for your organisation. Let’s explore them in detail now:
1. Data Objectives
First, you need to define clear goals and objectives for your data. The objectives should outline why you are collecting data and what you want to accomplish from analysing it. For example, “Employee Upskilling” is a suitable goal. This should be supported by a list of skills (and associated data points) that are valued by your organisation and measurable metrics related to upskilling.
2. Data Assimilation
One of the challenges L&D teams face is knowing where to find big data. But instead of losing time choosing the best sources, you should broaden your scope and collect all of the data that comes your way. Remember that you will still have the opportunity to narrow down the quantity of data later.
3. Find a Data Analysis Tool
Big data only gets bigger over time as your organisation grows. Therefore you need to have a big data analytics tool that is flexible and scalable.
In the eLearning industry, learning management systems (LMSs) are a very effective source of data to be analysed. Additionally, their analytics tools such as their reporting capabilities give you the ability to track learner progress and offer insights into their habits and behaviour.
4. Data Rationalisation
Not all of the data you collect will be relevant and actionable when it comes to your online learning programme. For instance, L&D teams are unlikely to be concerned with what brands or models of devices are used to access their content (unless there are compatibility issues).
Therefore, you should select and filter your data based on the goals and objectives you have set out in the first step.
5. Data Metrics
As mentioned above, big data comes in a variety of structures. A metric is a singular element of data processed or calculated based on data contained in the various structures. Data metrics allow you to separate and measure a certain aspect of your data.
You should use appropriate metrics to make sure your big data analysis is as effective as it can be. For instance, learning metrics may include: student progress/completion, assessment scores, experience points, proficiency levels and social club interactions.
6. Data Prioritisation
If you have several goals and objectives, it would be wise to create a priority list. This will help you focus on the most pertinent issues at hand. You will quickly establish which problems need to be addressed right away and which can be dealt with at a later stage. Issues that are preventing your learners from getting the full benefit of the learning programme should be taken care of as soon as possible.
For instance, a learning object that is too challenging can lead to learner frustration. In turn, this can lead to decreased motivation and engagement. However, learner feedback and LMS data analysis can help you identify problematic areas. You can then fine-tune your learning programmes to achieve better outcomes.
7. Data Protection
One of the most crucial aspects of managing vast amounts of big data is security. Big data is a valuable asset, which is why it always needs to be protected (especially if it contains personal information). You need to make sure you encrypt all data.
In addition, you should make sure that all of the connections are secure and that you have the necessary authentication protocol in place. Furthermore, you should keep an eye on who has access to your data and how they are using it.
The Challenges of Big Data Analytics
Big data systems are typically tailored to your specific needs. As such, designing the system architecture can be challenging. This is even more true when integrating an LMS system (and its data) into your big data analysis tool. This usually requires a custom-built solution. To pull this off, you need skill and experience that most database administrators and developers don’t have.
The capacities required in terms of storage and processing are rather large and tend to grow rapidly. As a result, the costs can quickly get out of hand even if (or especially if), you are using cloud-based solutions.
Using a cloud managed service can ease the design, storage and processing challenge, but may add limits in scope and customisation. Furthermore, migrating data sets and procedural processes from one platform to another, whether in-house or online, is also often a complex process.
Last but not least, making the data accessible to data analysts is a challenge when the data and processes reside on multiple processing and storage platforms. This is even truer when data variety and velocity are high.
Looking Ahead
However, in spite of new technologies bringing a fair share of new challenges, big data will continue to add value. Gathering information from various sources and analysing it helps you see the bigger picture.
It provides you with the necessary insights to make better decisions, know your employees and clients better, as well as understand the details of different and often complicated processes.
Moreover, if you don’t embrace big data, you risk getting left behind. NewVantage Partners have found that 97.2% of organisations are investing in big data and AI initiatives as they seek to become nimble, data-driven businesses. Don’t be part of the 2.8%!
Final Words
Big data analysis requires a substantial investment of time, money and energy. That being said, there is no better way to gain an understanding of what is happening within your organisation. It allows you to identify patterns which you would not have been able to see before. You can use these patterns to make better informed decisions for your business.
As author and consultant Geoffrey Moore puts it, “without big data analytics, companies are blind and deaf, wandering out onto the web like deer on a freeway”. The headlights of the future await us. It’s time to move forward with our eyes open, informed by data and ready to make the right decisions.
Once you have implemented all of these best practices, all you need to do is take steps to act on the resulting analysis. You can use your findings to tweak, update or revamp your learning strategies and fine-tune your individual courses or learning objects.
You may even be able to automate some of these steps. And once you’ve achieved that, there’s no limitation on the impact that your training interventions can have.
Are you interested in finding out how you can use data to drive engagement, improve learning initiative effectiveness and business impact? Contact us now to see the Impact Suite in action!
Unlimited Learner Engagement on
The Impact Suite
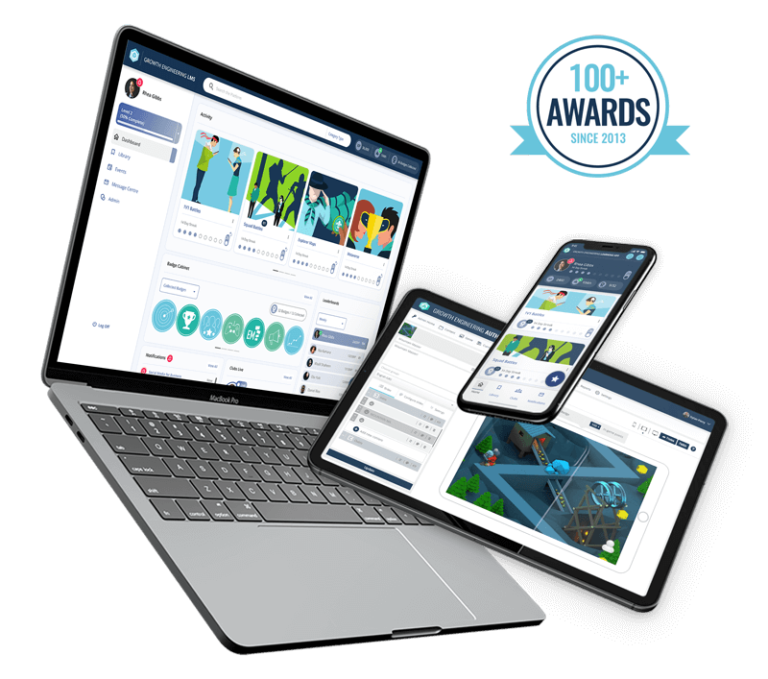